本記事はLangChain入門の4回目です。Chainについて説明します。Chainとは鎖という意味です。LangChainという名前から予想できるように、言語モデルを使った処理を鎖のようにつなげて実行する機能です。

それぞれの処理に入力と出力があり、ある処理の出力が次の処理の入力になってゆく、そんなイメージです。
本記事はFuture Coders独自教材からの抜粋です。更新の多い分野なので現状の内容と異なる場合があることご了承ください。
LangChain入門 – 4)Chains チェイン 目次
OpenAIを直接呼び出す場合、Chainを単体で実行する場合、それらを比較する例を以下に示します。
from langchain_openai import OpenAI
from langchain.prompts import PromptTemplate
from langchain.chains import LLMChain
llm = OpenAI()
# 1) LLM単体1
r = llm.invoke("日本の首都は?")
print(r)
## 2) LLM単体2
template = PromptTemplate.from_template("{place}の首都は?")
r = llm.invoke(template.format(place="日本"))
print(r)
## 3) Chain単体
chain = LLMChain(llm=llm, prompt=template, verbose=True)
r = chain.invoke("アメリカ")
print(r)
上記サンプルでは生成AIを3回実行しています。
出力は以下のようになりました。
東京
東京
> Entering new LLMChain chain...
Prompt after formatting:
アメリカの首都は?
> Finished chain.
{'place': 'アメリカ', 'text': '\n\nアメリカの首都はワシントンD.C.です。'}
最初は直接”日本の首都は?”と聞きました。OpenAIに直接プロンプトを渡しています。
2つ目はTemplateを使っていますが、template.formatを使って文字列に直しているだけで、1つ目と同じように文字列をOpenAIに渡しています。どちらも”東京です。”という答えが返ってきています。
3つ目がChainを使ったサンプルです。注目してほしいのは、LLMChainの引数にOpenAIのオブジェクト、プロンプトのテンプレートを渡していることです。つまり、LLMと”{place}の首都は?”というテンプレートのみをChainに渡しているのです。最後にchain.run(“アメリカ”)と実行していますが、明示的に「placeというパラメタに”アメリカ”をあてはめる」という旨は記述していません。
chainオブジェクトを作成したときにverbose=Trueと指定しているので、途中経過が出力されています。以下の範囲に含まれている箇所がChainの中の処理になります。
> Entering new LLMChain chain...
Prompt after formatting:
アメリカの首都は?
> Finished chain.
{'place': 'アメリカ', 'text': '\n\nアメリカの首都はワシントンD.C.です。'}
このChainでは、プロンプトに{place}というパラメタがあり、chain.run(“アメリカ”)と実行することで、placeというパラメタには”アメリカ”という値を当てはめるものと判断して処理を進めていることがわかります。
直接OpenAIを呼び出したときと、Chainを使った時の違いのイメージを以下に示します。
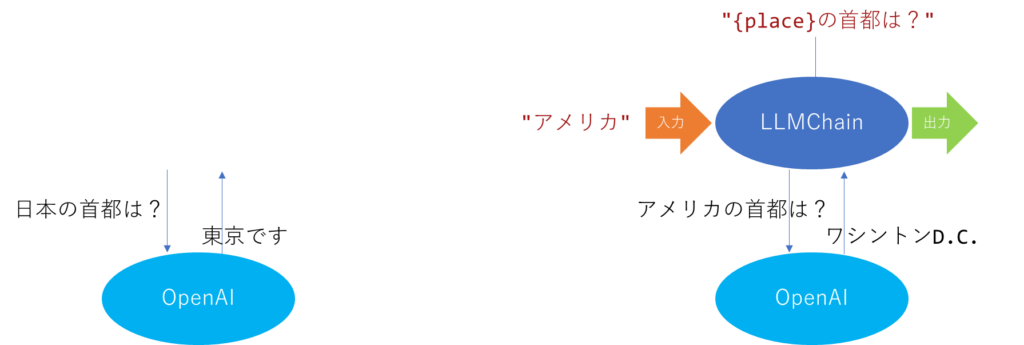
Chainは単体で使ってもありがたみはありません。では鎖をつなげてみるとどんなことが可能になるか見てゆきましょう。
SimpleSequentialChain
SimpleSequentialChainは処理を順番につなげてゆくときに使用するクラスです。
たとえば、以下のような2ステップからなる作業を考えます。
- 会社の名前を考える
- その名前をベースにキャッチフレーズのアイディアをだす
最初の処理を実行し、その結果を次の処理へ入力する、処理の結果をつなげて実行するイメージです。
例を見てみましょう。
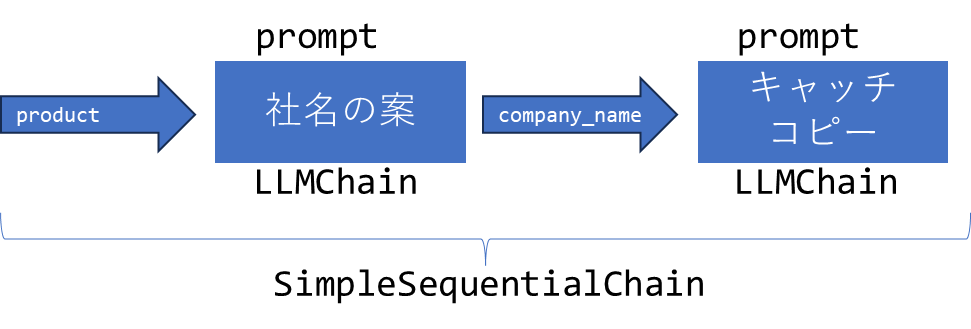
from langchain_openai import OpenAI
from langchain.prompts import PromptTemplate
from langchain.chains import LLMChain, SimpleSequentialChain
llm = OpenAI()
prompt_1st = PromptTemplate(
input_variables=["product"],
template="{product}を作る会社の社名の案を1つ教えてください。",
)
print(prompt_1st)
chain_1st = LLMChain(llm=llm, prompt=prompt_1st)
prompt_2nd = PromptTemplate(
input_variables=["company_name"],
template="{company_name}という会社名のキャッチコピーを考えてください。",
)
chain_2nd = LLMChain(llm=llm, prompt=prompt_2nd)
sequential_chain = SimpleSequentialChain(chains=[chain_1st, chain_2nd], verbose=True)
r = sequential_chain.invoke("風鈴")
print(r)
タスク1つをLLMChainオブジェクトで表現して、SimpleSequentialChainでそれらをつなげています。
SimpleSequentialChainの引数において、verbose=Trueとすることで、途中経過も出力されます。
出力は以下のようになりました。
> Entering new SimpleSequentialChain chain...
「鳴風堂」
「心に響く、風のようなおいしさ」
> Finished chain.
{'input': '風鈴', 'output': '\n\n「心に響く、風のようなおいしさ」'}
社名案が表示され、そのキャッチコピーが出力されていることがわかります。プログラムを作成している段階では、どのような社名が候補として列挙されるかわかりません。前段の処理結果を受け取って処理するために、パラメタ化されたテンプレートを使用する必要があることに注意してください。
ちなみに、テンプレートを作成するコードはPromptTempalate.from_templateを使ってもかまいません。同じ結果が得られます。
prompt_1st = PromptTemplate.from_template(
"{product}を作る会社の社名の案を3つ教えてください。"
)
chain_1st = LLMChain(llm=llm, prompt=prompt_1st)
prompt_2nd = PromptTemplate.from_template(
"{company_name}という会社名のキャッチコピーを考えてください。",
)
chain_2nd = LLMChain(llm=llm, prompt=prompt_2nd)
連鎖は2つ以上でも大丈夫です。以下は3つのタスクを連鎖させている例です。
from langchain_openai import OpenAI
from langchain.prompts import PromptTemplate
from langchain.chains import LLMChain, SimpleSequentialChain
llm = OpenAI(temperature=0.1)
prompt_1st = PromptTemplate.from_template(
"{city}近郊の日帰りに最適な場所を1つ教えてください。"
)
chain_1st = LLMChain(llm=llm, prompt=prompt_1st)
prompt_2nd = PromptTemplate.from_template(
"{place}で人気のグルメを1つ教えてください。",
)
chain_2nd = LLMChain(llm=llm, prompt=prompt_2nd)
prompt_3rd = PromptTemplate.from_template(
"{food}の値段の相場を教えてください",
)
chain_3rd = LLMChain(llm=llm, prompt=prompt_3rd)
sequential_chain = SimpleSequentialChain(chains=[chain_1st, chain_2nd, chain_3rd], verbose=True)
r = sequential_chain.invoke("東京")
print(r)
出力は以下のようになりました。
> Entering new SimpleSequentialChain chain...
東京近郊の日帰りに最適な場所としては、神奈川県の鎌倉がおすすめです。鎌倉は東京から約1時間程度の距離にあり、歴史的な建造物や美しい自然が楽しめる観光スポットが多くあります。また、鎌倉は海に面しているため、海水浴やマリンスポーツも楽しむことができます。さらに、鎌倉は多くのお店やレストランが集まる小町通りや、おしゃれなカフェが並ぶ江ノ島など 、食やショッピングも楽しめる場所がたくさんあります。日帰りで気軽に訪れることができる鎌倉は、東京近郊のおすす
鎌倉でおすすめのグルメとしては、鎌倉駅近くにある「鎌倉小町」が挙げられます。こちらは鎌倉名物の「小町饅頭」を販売しているお店で、鎌倉小町の名前の由来にもなっています。小 町饅頭は、小豆餡を包んだもちもちとした生地が特徴的で、鎌倉のお土産としても人気があります。また、鎌倉小町では小町饅頭のほかにも、季節限定の饅頭や和菓子、抹茶スイーツなど も販売しており、鎌倉の魅力を詰め込んだお店として人気を集めています。鎌
鎌倉の値段の相場は、一般的な観光地と比べるとやや高めです。例えば、鎌倉の有名な観光スポットである鶴岡八幡宮の入場料は200円、大仏殿の入場料は200円、鎌倉宮の入場料は300円などとなっています。また、鎌倉のお土産として人気のある小町饅頭の値段は、1個100円程度から販売されています。飲食店の場合は、ランチで1000円程度から、ディナーで3000円程度から となっています。ただし、季節や店舗によって値段は異なるため、具体的な相場は変動する可能性があります。
> Finished chain.
{'input': '東京', 'output': '\n\n鎌倉の値段の相場は、一般的な観光地と比べるとやや高めです。例えば、鎌倉の有名な観光スポットである鶴岡八幡宮の入場料は200円、大仏殿の入場 料は200円、鎌倉宮の入場料は300円などとなっています。また、鎌倉のお土産として人気のある小町饅頭の値段は、1個100円程度から販売されています。飲食店の場合は、ランチで1000円 程度から、ディナーで3000円程度からとなっています。ただし、季節や店舗によって値段は異なるため、具体的な相場は変動する可能性があります。'}
ConversationChain
ConversationChainは会話処理を行うためのモデルです。会話を扱うのでOpenAIではなく、ChatOpenAIを使用している点に注意してください。
シンプルな例をみてみましょう。
from langchain.chains import ConversationChain
from langchain_openai import ChatOpenAI
chain = ConversationChain(llm=ChatOpenAI())
s = input("> ")
r = chain.invoke(s)
print(r)
s = input("> ")
r = chain.invoke(s)
print(r)
以下実行結果です。
> こんにちは
こんにちは!お元気ですか?何かお手伝いできることはありますか?
> 東京の夏は暑いですね
はい、東京の夏は非常に暑くなります。平均気温は30度以上になることも多く、湿度も高いです。特に7月から8月にかけては、蒸し暑さが厳しく感じ
られます。外出する際は、涼しい服装や帽子、日焼け止めなどの対策が必要です。また、エアコンや扇風機を利用することも一般的です。夏の暑さに
は十分に気を付けてくださいね。
for文を使って繰り返し会話するように修正してみましょう。以下サンプルです。ENTERが入力されるまで会話を繰り返します。
from langchain.chains import ConversationChain
from langchain_openai import ChatOpenAI
chain = ConversationChain(llm=ChatOpenAI(), verbose=True)
s = input("> ")
while s != "":
r = chain.invoke(s)
print(r)
s = input("> ")
以下「こんにちは、私は太郎、8歳です。」「私は来年何歳になるでしょうか?」と入力したときの出力例です。 verbose=Trueを指定しているので、Chainの中で何が行われているかも出力されています。
> こんにちは、私は太郎、8歳です。
> Entering new ConversationChain chain...
Prompt after formatting:
The following is a friendly conversation between a human and an AI. The AI is talkative and provides lots of specific details from its context. If the AI does not know the answer to a question, it truthfully says it does not know.
Current conversation:
Human: こんにちは、私は太郎、8歳です。
AI:
> Finished chain.
こんにちは太郎さん!私はAIです。どのようにお手伝いできますか?
> 私は来年何歳になるでしょうか?
> Entering new ConversationChain chain...
Prompt after formatting:
The following is a friendly conversation between a human and an AI. The AI is talkative and provides lots of specific details from its context. If the AI does not know the answer to a question, it truthfully says it does not know.
Current conversation:
Human: こんにちは、私は太郎、8歳です。
AI: こんにちは太郎さん!私はAIです。どのようにお手伝いできますか?
Human: 私は来年何歳になるでしょうか?
AI:
> Finished chain.
太郎さん、あなたが現在8歳であると言われましたので、来年は9歳になります。
ここで注目してほしいのは、最初に名前と年齢を伝えたら、そのことを覚えていた(来年は9歳と答えた)ということです。その理由はChainの中で何がおきていたか見るとよく分かります。人の入力、AIからの応答、すべてメッセージの中に含まれていることが分かります。これによって、会話のコンテキストが保存されていたのです。
以下はStreamlitを使ってアプリ化したサンプルです。
import streamlit as st
from langchain.chains import ConversationChain
from langchain_openai import ChatOpenAI
if "chat" not in st.session_state:
st.session_state["chat"] = ConversationChain(llm=ChatOpenAI(), verbose=True)
if "msg" not in st.session_state:
st.session_state["msg"] = []
chain = st.session_state["chat"]
st.title("Chatbot by LangChain")
for msg in st.session_state["msg"]:
with st.chat_message(msg["role"]):
st.markdown(msg["content"])
if prompt := st.chat_input(""):
with st.chat_message("user"):
st.markdown(prompt)
response = chain.invoke(prompt)
with st.chat_message("assistant"):
st.markdown(response["response"])
st.session_state["msg"].append({"role":"user", "content":prompt})
st.session_state["msg"].append({"role":"assistant", "content":response})
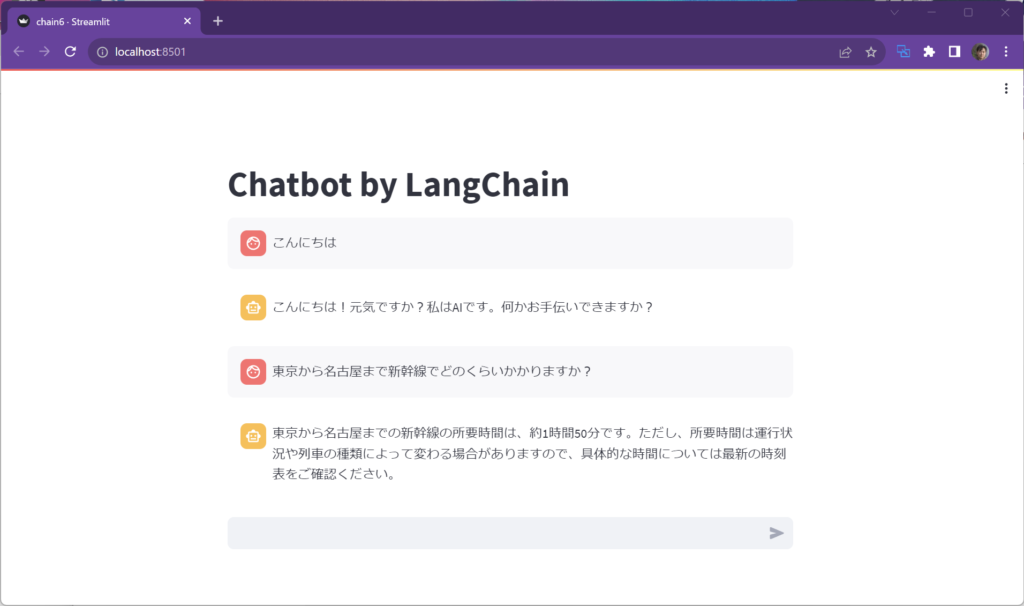
このように簡単に会話をするアプリを作成できるようになりました。コンソールをみると途中経過が出力されています。入力した会話が全て保存されていることが分かります。
> Entering new ConversationChain chain...
Prompt after formatting:
The following is a friendly conversation between a human and an AI. The AI is talkative and provides lots of specific details from its context. If the AI does not know the answer to a question, it truthfully says it does not know.
Current conversation:
Human: こんにちは
AI: こんにちは!元気ですか?私はAIです。何かお手伝いできますか?
Human: 東京から名古屋まで新幹線でどのくらいかかりますか?
AI:
> Finished chain.
このことから気づいた方もいるかもしれません。会話が長く続くほど、処理するテキストが長くなってゆきます。そのうちトークンの上限に到達すると会話が途切れてしまうでしょう。会話が長引くほど処理コストがかかってしまうことも問題です。
人間の会話においても、一字一句間違えずに覚えてることはないでしょう。「なんとなくこんなことを話した」とその内容を要約しながら覚えているはずです。LangChainにはそのような機能を実装するMemoryモジュールが用意されています。Memoryモジュールに関して詳しくは後述します。
Chainには他にも
- 入力を加工して出力するTransformChain
- 独自で繋がりを指定できるカスタムChain
- 動的に次の処理を選択するRouterChain
などが用意されています。詳しくは以下のURLを参照してください。
https://python.langchain.com/docs/modules/chains/
演習
chain-ex1.py
以下のChainを作成して生成AIで回答を求めてください。
- 東京から6時間以内で行けるおすすめのビーチを1つ
- その国名と人口を調べて
- その国のおすすめ料理を表示する
以下出力例です。
> Entering new chain...
サイパン島(Saipan Island)
マリアナ諸島(Commonwealth of the Northern Mariana Islands)、人口52,344人(2020年)
代表的な料理として、「チキンケータレット」があります。チキンケータレットは、マリアナ諸島の伝統的な料理で、チキン、ライス、野菜などを炒めたものです。
> Finished chain.
代表的な料理として、「チキンケータレット」があります。チキンケータレットは、マリアナ諸島の伝統的な料理で、チキン、ライス、野菜などを炒めたものです。
chain-ex2.py
日本とアメリカそれぞれで
- おすすめの都市
- その理由
を出力してください。以下出力例です。
日本では東京は、多様な文化を体験できる多国籍な都市です。
アメリカではニューヨークは、文化、美術、食文化、娯楽など、多くの要素が揃っているため、住むのに最適な都市です。
解答例
chain-ex1.py
from langchain_openai import OpenAI
from langchain.prompts import PromptTemplate
from langchain.chains import LLMChain, SimpleSequentialChain
llm = OpenAI(temperature=0.3)
prompt_1st = PromptTemplate.from_template(
"{city}から6時間以内のフライトで行けるおすすめの国外のビーチを1つ教えてください。\nビーチ名:"
)
chain_1st = LLMChain(llm=llm, prompt=prompt_1st)
prompt_2nd = PromptTemplate.from_template(
"{beach}がある国とその国の人口を教えてください。\n国名と人口:",
)
chain_2nd = LLMChain(llm=llm, prompt=prompt_2nd)
prompt_3rd = PromptTemplate.from_template(
"その国:{country}のおすすめ料理はなんですか?代表的な料理を1つ教えてください。",
)
chain_3rd = LLMChain(llm=llm, prompt=prompt_3rd)
sequential_chain = SimpleSequentialChain(chains=[chain_1st, chain_2nd, chain_3rd], verbose=True)
r = sequential_chain.invoke("東京")
print(r)
chain-ex2.py
from langchain_openai import OpenAI
from langchain.prompts import PromptTemplate
from langchain.chains import LLMChain, SimpleSequentialChain
llm = OpenAI(temperature=0.3)
prompt_1st = PromptTemplate.from_template(
"{country}で一番住むのにおすすめなのはどの都市ですか?"
)
chain_1st = LLMChain(llm=llm, prompt=prompt_1st)
prompt_2nd = PromptTemplate.from_template(
"{city}に住むのをおすすめする理由を1つ簡潔に教えてください。",
)
chain_2nd = LLMChain(llm=llm, prompt=prompt_2nd)
for place in ["日本", "アメリカ"]:
sequential_chain = SimpleSequentialChain(chains=[chain_1st, chain_2nd])
r = sequential_chain.invoke(place)
print(f"{place}では{r['output'].strip()}")